Introduction/Background
The customer team was challenged with creating a successful protocol trial design that would achieve the target end point for a phase 3 study involving a novel antibody-drug conjugate (ADC).
QuantHealth's ability to model the bio-pharmacological representation of novel drugs via a robust biomedical knowledge graph and simulate the impact of such novel treatments using AI models trained on very large clinical datasets, encouraged the team to explore this technology to run thousands of trials in silico to help define the optimum protocol design.
The customer chose to partner with QuantHealth over internal capabilities and other vendors due to:
Depth of data - A GenAI platform harnessing and combining data from >350M patients and >100,000 drug entities
Platform - An advanced analytics module used by the data science team to simulate novel clinical scenarios for driving insights and prospective evidence generation.
Customer support - A consulting and support team that provided the necessary training and guidance to enable the oncology disease team
Methodology
An initial sponsor protocol was simulated within a drug-specific model to evaluate expected primary outcome result both at interim analysis (IA2) and final analysis (FA). QuantHealth simulated >1,000 protocol variations – including potential target populations, tumor subtypes, comparator arms, duration of trial, sample size, and geographic areas of enrollment, to determine which factors were most likely to contribute to clinical and operational success.
Results
Exploring simulated subgroup generated insights for protocol optimization and trial de-risking, while balancing the impact of restricting the target population against with potential post-approval market size. Simulation allowed establishing high probability of success given the optimal protocol and planned sample size.
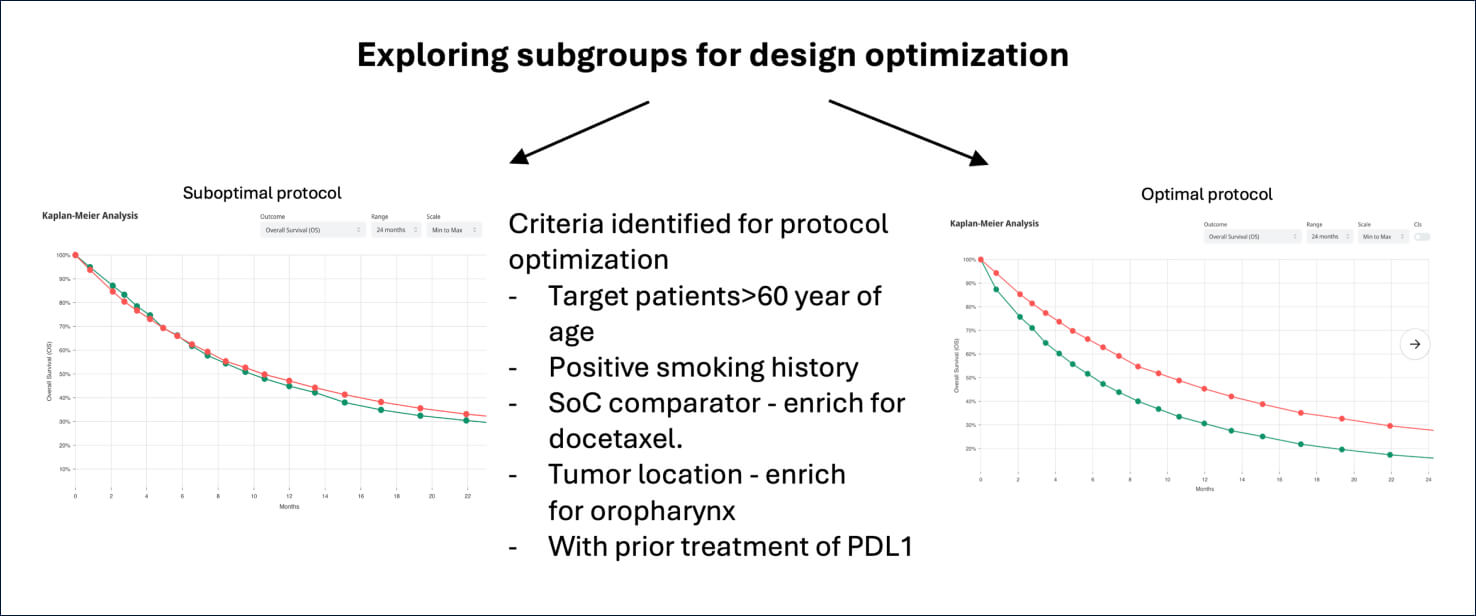
Net Benefits
Optimized protocol led to following gains:
1. More likely to hit 3-month improvement in median OS - favorably impacting probability of technical success, regulatory approval, and reimbursement.
2. More likely to cross efficacy boundary at preplanned interim analysis - shortening trial duration and time to market
Incremental Financial Return |
---|
$256 million – salvaging phase 3 trial |
$146 million - reduced time to market and increased time in peak sales by 8 months. |